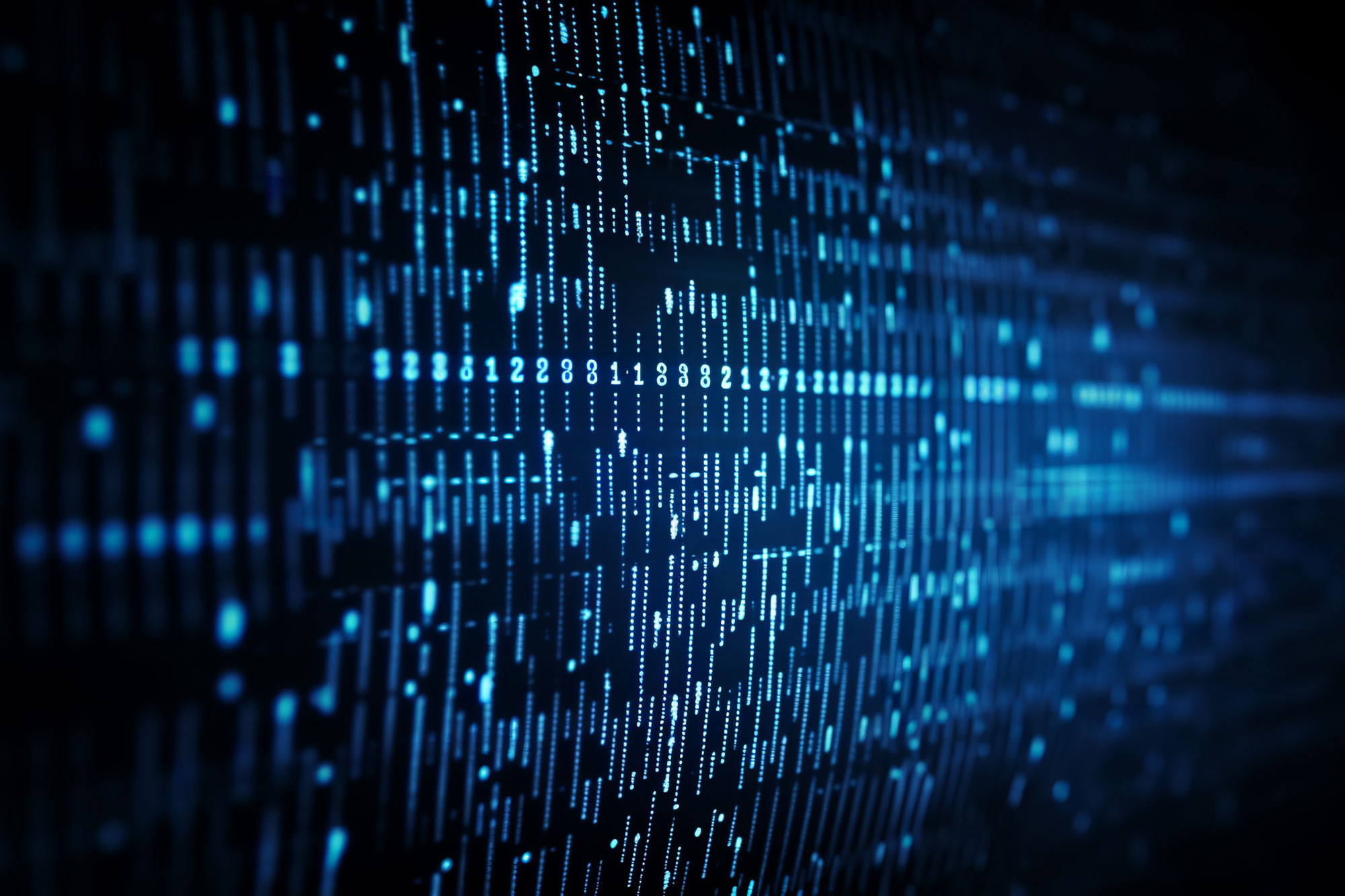
Academic staff
Professor Shahar Mendelson is a world leader in statistical learning theory and in the connections between high dimensional statistics, statistical learning theory, empirical processes, and asymptotic geometric analysis.
In his career, Professor Mendelson has authored numerous seminal articles. In his 2003 paper in Inventiones Mathematicae, he provided a complete solution to Talagrand's entropy problem. This article was followed by many others, reporting equally trailblazing results – for instance, a complete solution in the subgaussian setup to the approximate and the exact reconstruction problems of a sparse signal using few linear measurements, which was a significant extension of the renowned work of E. Candes and Fields Medallist T. Tao. Professor Mendelson and colleagues subsequently studied the recovery of sparse signals using a small number of measurements selected from certain structured measurement ensembles, making the recovery computationally feasible. Breakthrough results in the study of statistical problems in heavy-tailed situations followed. Most notably, optimal mean and covariance estimation, and the optimal accuracy-confidence trade-off in learning problems (with respect to the squared loss and under minimal assumptions). The latter was the most important unsolved problem in Statistical Learning Theory since the late 1960s.
Professor Peter Bartlett is a pioneer in statistical learning theory, which is at the interface of computer science and statistics, and is focused on the science behind large, complex statistical decision problems. He has created the theoretical foundations for many key advances in statistical machine learning.
Professor Bartlett’s contributions include analysing large margin classifiers (a successful family of computationally efficient methods for classifying patterns), developing and analysing statistical learning methods based on convex optimisation, and developing new techniques for analysing the performance of prediction methods.
He is the co-author, with Martin Anthony, of the book Neural Network Learning: Theoretical Foundationsrefxs. He has served as an associate editor of the journals Bernoulli, Mathematics of Operations Research, the Journal of Artificial Intelligence Research, the Journal of Machine Learning Research, the IEEE Transactions on Information Theory, Machine Learning, and Mathematics of Control Signals and Systems, and as program committee co-chair for COLT and NIPS. He has consulted to a number of organizations, including General Electric, Telstra, SAC Capital Advisors, and Sentient.