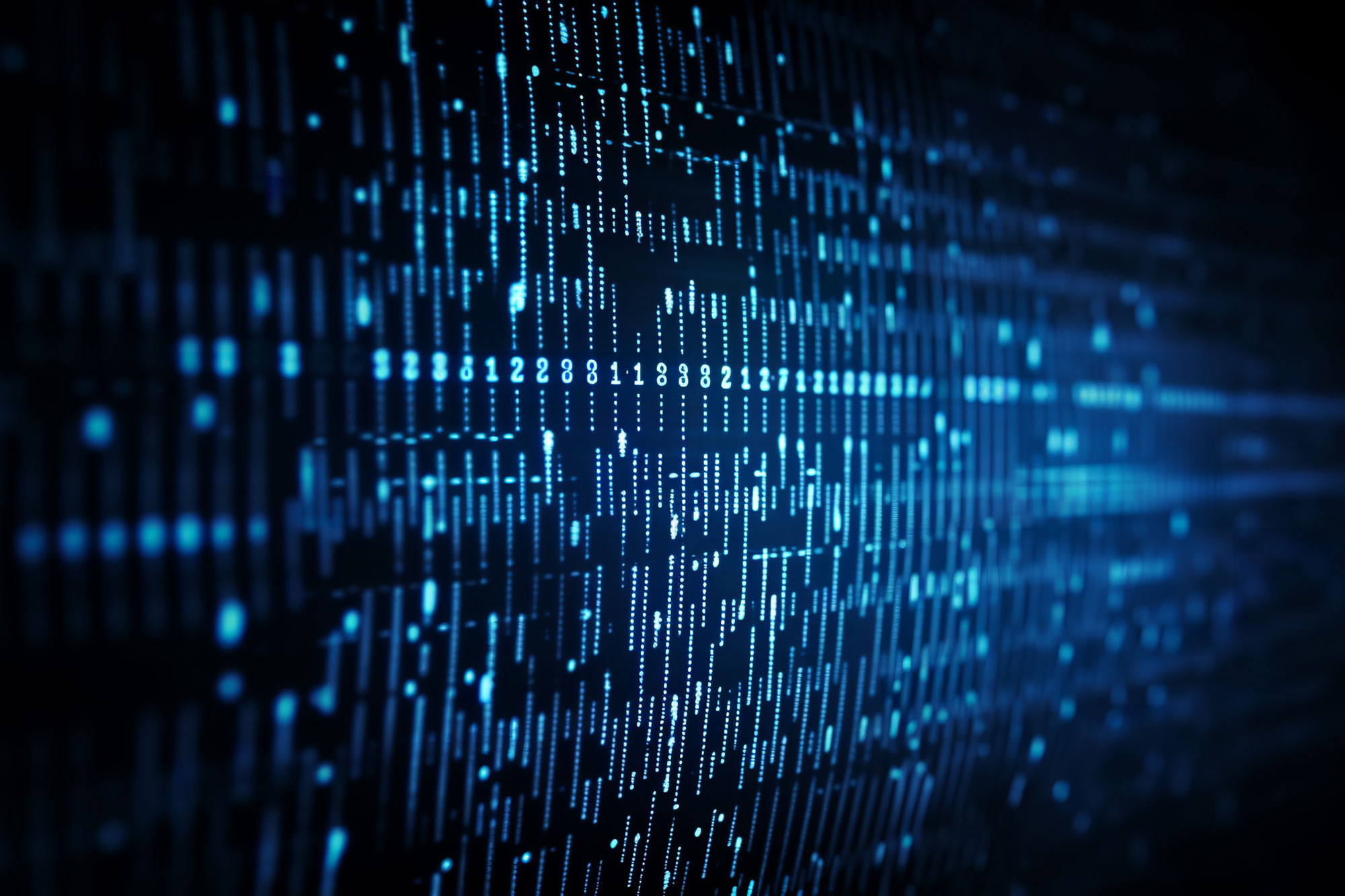
Annual Graduate School in Mathematical Aspects of Data Science
The first Global School Series in Mathematical Aspects of Data Science, hosted by the Mathematical Sciences Institute (MSI), Australian National University (ANU) at the Darwin Convention Centre. Intended for graduate level students and centred on the mathematical foundations of data science.
About
About
The first Global School Series in Mathematical Aspects of Data Science will be held from Mon, Jun 24 2024 to Fri, Jun 28 2024 at the Darwin Convention Centre, in Darwin (Northern Territory, Australia. The aim of the series is to expose graduate students and early-career researchers to the beautiful and exciting mathematical theory that is needed in modern data science research.
The realisation of this Winter School is facilitated via a generous donation by Google Research Australia. The Google donation will be used to assist the participation of staff and students to our inaugural Winter School. Please register your participation as soon as possible to avoid disappointment, since the number of places is limited.
This year, the school will consist of short courses presented by:
Peter Bartlett (University of California Berkeley and Google DeepMind)
Subhro Ghosh (National University of Singapore)
Gabor Lugosi (Pompeu Fabra University Barcelona)
Shahar Mendelson (Australian National University)
Jonathan Scarlett (National University of Singapore)
Nikita Zhivotovskiy (University of California Berkeley)
Travel Grants
Multiple 500.00AUD per participant Travel Grants are available for Australian Citizens studying at Australian Universities. Apply for funding now.
Scientific Committee
Gabor Lugosi (Pompeu Fabra University Barcelona), Shahar Mendelson (Australian National University), Roman Vershynin (University of California Irvine), Peter Bartlett (University of California Berkeley and Google Research)
Venue
Darwin Convention Centre
10 Stokes Hill Rd
Darwin City NT 0800
Australia